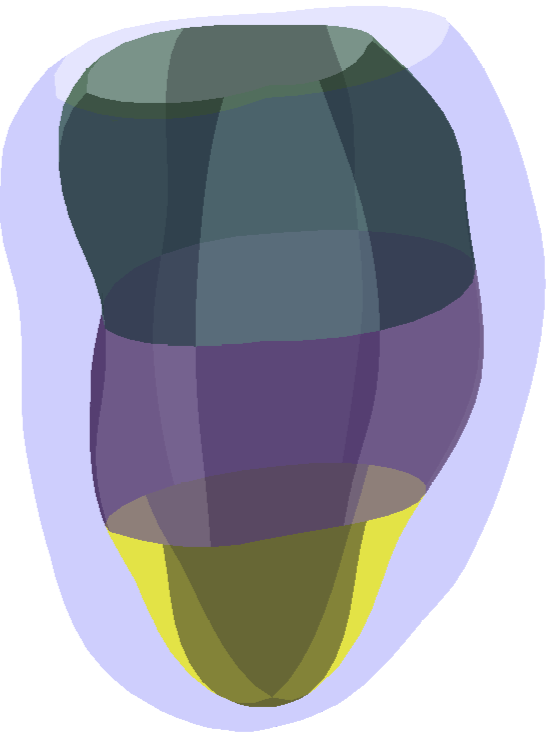
In order to maintain normal cardiac physiology, the heart constantly changes its shape and function (remodelling) due to myocardial injury, disease or excessive physical activities. Myocardial infarction does affected the shape of the heart due to scars or reduced contractility. At the early stage of infarction, wall stress increases due to infarct expansion forcing the heart muscle to dilate (increasing the cavity volume). The ejection fraction was still near normal until prolonged infarction causes more insidious process when the compensatory remodelling fails to maintain the cardiac output (loss of myocytes, reduction of ejection fraction and increasing the systolic volume). Can these myocardial infarction remodelling be detected automatically from the 3D model of the left ventricle?
At STACOM 2015, we organised a challenge to automatically detect left ventricular shapes of patients with myocardial infarction from asymptomatic subjects. This challenge attracted 11 research groups worldwide with different ways to extract optimal features from the left ventricular shapes and varying machine learning algorithms, including random forests, support vector machines and point distribution models.
Participants
These are participants of STACOM 2015. Read more from [Suinesiaputra et al., 2017].
- SSM-PLS: Statistical Shape Modeling Using Partial Least Squares: Application to the Assessment of Myocardial Infarction
K. Lekadir, X. Albà, M. Pereañez, and A. F. Frangi - GMPT: Systo-Diastolic LV Shape Analysis by Geometric Morphometrics and Parallel Transport Highly Discriminates Myocardial Infarction
P. Piras, L. Teresi, S. Gabriele, A. Evangelista, G. Esposito, V. Varano, C. Torromeo, P. Nardinocchi, and P. E. Puddu - IC-ShapeMotion: Classification of Myocardial Infarcted Patients by Combining Shape and Motion Features
W. Bai, O. Oktay, and D. Rueckert - MS: Detecting Myocardial Infarction Using Medial Surfaces
P. Ablin and K. Siddiqi - ASMSVM: Left Ventricle Classification Using Active Shape Model and Support Vector Machine
N.Parajuli, A.Lu, and J.S.Duncan - FM: Supervised Learning of Functional Maps for Infarct Classification
A. Mukhopadhyay, I. Oksuz, and S. A. Tsaftaris - JCCA: Joint Clustering and Component Analysis of Spatio-Temporal Shape Patterns in Myocardial Infarction
C. Pinto, S. Cįmen, A. Gooya, K. Lekadir, and A. F. Frangi - RF: Myocardial Infarction Detection from Left Ventricular Shapes Using a Random Forest
J. Allen, E. Zacur, E. Dall’Armellina, P. Lamata, and V. Grau - PT: Combination of Polyaffine Transformations and Supervised Learning for the Automatic Diagnosis of LV Infarct
M.-M. Rohé, N. Duchateau, M. Sermesant, and X. Pennec - HeAT-RDF: Automatic Detection of Cardiac Remodeling Using Global and Local Clinical Measures and Random Forest Classification
J. Ehrhardt, M. Wilms, H. Handels, and D. Säring - L2GF: Automatic Detection of Myocardial Infarction Through a Global Shape Feature Based on Local Statistical Modeling
M. Tabassian, M. Alessandrini, P. Claes, L. Marchi, D. Vandermeulen, G. Masetti, and J. D’hooge
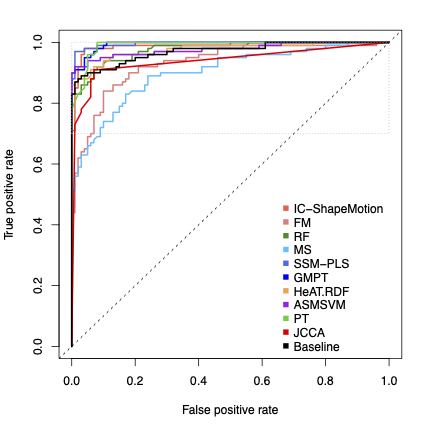
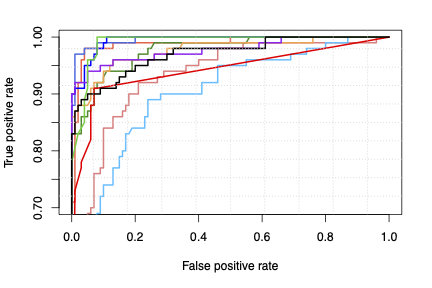
Data
The training dataset will comprise one hundred (100) cases with myocardial infarction and an additional one hundred (100) asymptomatic cases from the DETERMINE and MESA datasets respectively, contributed to the Cardiac Atlas Project (www.cardiacatlas.org).
Shapes will be provided as corresponding Cartesian point sets in cardiac MRI magnet coordinates at end-diastole (ED) and end-systole (ES). Classification labels indicating disease (0 = normal, 1 = infarcted) will be provided for the training dataset. No images will be provided.
The goals are to:
- Establish a statistical shape model from the set of 3D shapes
- Classify cases between normal or abnormal (with myocardial infarct)
The participants’ methods will be tested in a different set of 200 cases, again containing 100 asymptomatic cases and 100 infarcted cases. Classification accuracy and related measures of agreement will be calculated (specificity, sensitivity, etc.).
It is expected that the probabilistic models can be easily visualised but there is no restriction on the type of decomposition used to partition the shape space (i.e. can be linear or non-linear). Both supervised and non-supervised classification methods can be submitted (if there are enough of both a comparison might be made). A peer-reviewed paper summarising the findings of this challenge will be submitted to an appropriate journal.