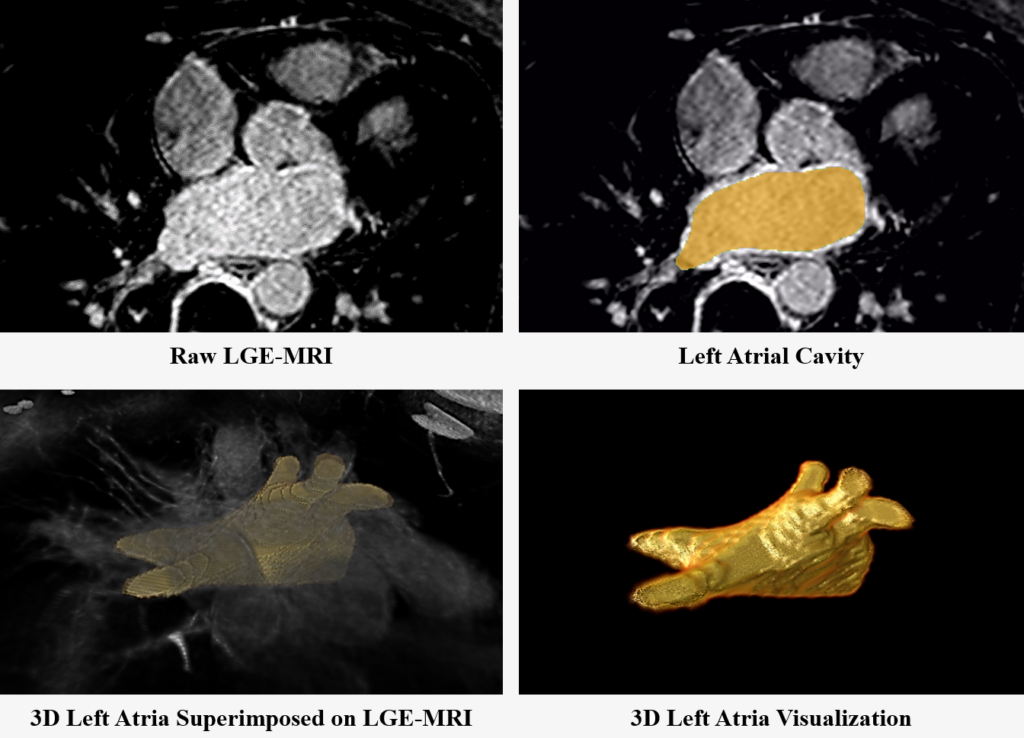
Atrial fibrillation (AF) is the most common type of cardiac arrhythmia. The poor performance of current AF treatment is due to a lack of understanding of the structure of the human atria.
Nowadays, gadolinium contrast agencies are used in a third of all MRI scans to improve the clarity of the images of a patient's internal structures, such as the atria. Gadolinium-enhanced magnetic resonance imaging (GE-MRI) is widely used to study the extent of fibrosis (scars) across the atria [2]. Recent studies on human atria imaged with GE-MRI have suggested fingerprints of the atrial structure may hold the key to understanding and reversing AF [3][4].
Direct segmentation of the atrial chambers from GE-MRIs is very challenging due to the low contrast between the atrial tissue and background. Most of the existing atrial structural analysis studies utilizing GE-MRIs have been based on labor-intensive, error/bias-prone manual segmentation. Hence, there is a need for an intelligent algorithm that can perform fully automatic atrial segmentation for the left atrial (LA) cavity, to accurately reconstruct and visualize the atrial structure for clinical usage [5].
This challenge has provided an open competition for wider communities to test and validate their methods for image segmentation on a large 3D clinical dataset. The exciting development is a very important step towards patient-specific diagnostics and treatment of AF.
The benchmarking study which summarizes this challenge is now available, published in Medical Image Analysis [1] [link here].
Data Summary
Size | 100 Data for Training |
54 Data for Testing | |
Pathology | Atrial Fibrilation |
Images | 3D Gadolinium-Enhanced Magnetic Resonance Imaging |
Links | 3D Binary Masks of the Left Atrial Cavity |
The Task
The participants are required to produce a computational framework capable of performing fully automatic segmentation of the LA cavity from 3D GE-MRIs without any manual assistance. The participants will receive 100 data+masks to develop their approach and be evaluated on 54 different test data for evaluation. The participants will submit the masks of the LA cavity for the 54 patients. The evaluation will be done by comparing the submitted masks for the test data the with their manually segmented masks (not open to the public). The test data (data only) will be released 2 weeks prior to the deadline of the challenge so participants can submit their predicted masks. This challenge provides a chance for participants to carefully study and experiment on a large GE-MRI dataset, and further push the state-of-the-art performance for atria segmentation.
This challenge was held in STACOM 2018 workshop and has been closed. You may download the training and testing data, but you cannot submit it for prediction.
To download the dataset and other relevant files, please visit the Data section of the challenge.
To submit your predictions for the challenge, please visit the Submission section of the challenge.
Final Rankings
Prizes awarded:
- The team in 1st place will receive an NVIDIA GPU as an award
- The team in 2nd place will receive $300 USD.
- The team in 3rd place will receive $200 USD.
- The top performers will also be invited to co-author in an international benchmarking study.
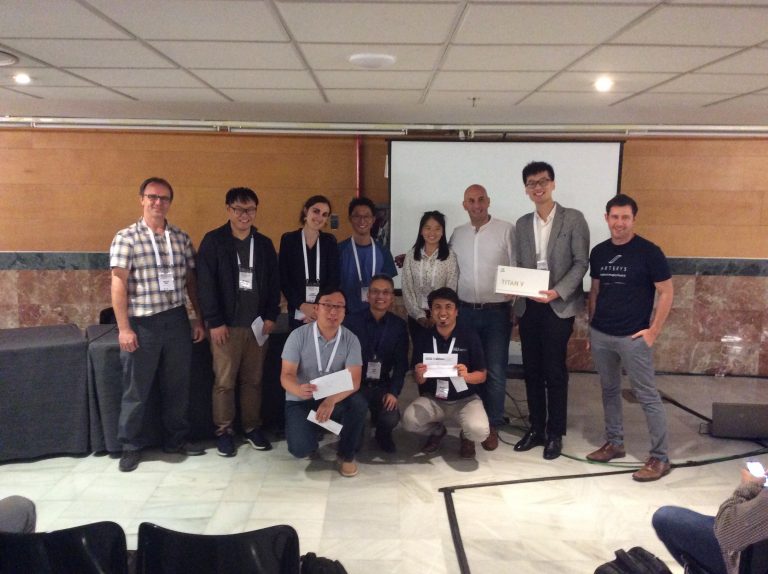
The final rankings for the top 15 out of 18 conference attending teams
Rank | Author | Score | |
1 | Qing Xia, Yuxin Yao, Zhiqiang Hu, Aimin Hao | 0.932 | |
2 | Cheng Bian, Xin Yang, Jianqiang Ma, Shen Zheng, Yu-An Liu, Reza Nezafat, Pheng-Ann Heng, Yefeng Zheng | 0.926 | |
2 | Sulaiman Vesal, Nishant Ravikumar, and Andreas Maier | 0.926 | |
3 | Caizi Li, Qianqian Tong, Xiangyun Liao, Weixin Si, Yinzi Sun, Qiong Wang, Pheng Ann Heng | 0.923 | |
3 | Elodie Puybareau, Zhao Zhou, Younes Khoudli, Yongchao Xu, Jerome Lacotte, Thierry Geraud | 0.923 | |
3 | Xin Yang, Na Wang, Yi Wang, Xu Wang, Reza Nezafat, Dong Ni, Pheng-Ann Heng | 0.923 | |
4 | Chen Chen, Wenjia Bai, and Daniel Rueckert | 0.921 | |
5 | Shuman Jia, Antoine Despinasse, Zihao Wang, Herve Delingette, Xavier Pennec, Pierre Jaıs, Hubert Cochet, and Maxime Sermesant | 0.907 | |
6 | Yashu Liu, Yangyang Dai, Cong Yan, Kuanquan Wang | 0.903 | |
7 | Davide Borra, Alessandro Masci, Lorena Esposito, Alice Andalo, Claudio Fabbri, Cristiana Corsi | 0.898 | |
8 | Coen de Vente, Mitko Veta, Orod Razeghi, Steven Niederer, Josien Pluim, Kawal Rhode, Rashed Karim | 0.897 | |
9 | Chandrakanth Jayachandran Preetha, Shyamalakshmi Haridasan, Vahid Abdi, Sandy Engelhardt | 0.888 | |
10 | Mengyun Qiao, Yuanyuan Wang, Rob J. van der Geest, Qian Tao | 0.862 | |
11 | Marta Nunez-Garcia, Xiahai Zhuang, Gerard Sanroma, Lei Li, Lingchao Xu, Constantine Butakoff, Oscar Camara | 0.859 | |
12 | Nicolo Savioli, Giovanni Montana, Pablo Lamata | 0.851 |
The final rankings for the top 22 out of a total of 27 participants
Rank | Author | Score |
1 | Qing Xia et al. | 0.932 |
2 | Ning Huang | 0.931 |
3 | Xinlong Sun | 0.930 |
4 | Cheng Bian et al. | 0.926 |
4 | Px Zhan | 0.926 |
4 | Sulaiman Vesal et al. | 0.926 |
5 | Caizi Li et al. | 0.923 |
5 | Elodie Puybareau et al. | 0.923 |
5 | Xin Yang et al. | 0.923 |
6 | Chen Chen et al. | 0.921 |
6 | Ed | 0.921 |
7 | Qinyi Zhang | 0.920 |
8 | Lingchao Xu | 0.915 |
9 | Shuman Jia et al. | 0.907 |
10 | Xiaochuan Li | 0.904 |
11 | Yashu Liu et al. | 0.903 |
12 | Davide Borra et al. | 0.898 |
13 | Coen de Vente et al. | 0.897 |
14 | Chandrakanth Jayachandran Preetha et al. | 0.888 |
15 | Mengyun Qiao et al. | 0.862 |
16 | Marta Nunez-Garcia et al. | 0.859 |
17 | Nicolo Savioli et al. | 0.851 |
Organizers
The challenge is proudly sponsored by:
- Nvidia Corporation (United States)
- The MedTech Centre of Research Excellence (New Zealand)
- Arterys (Medical Imaging Cloud AI)
- NewRealReview.com (United States)
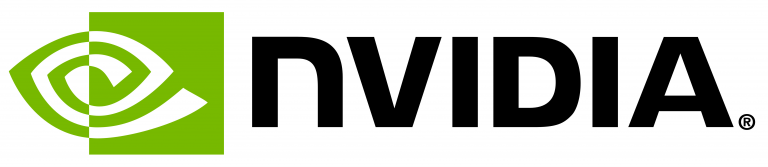
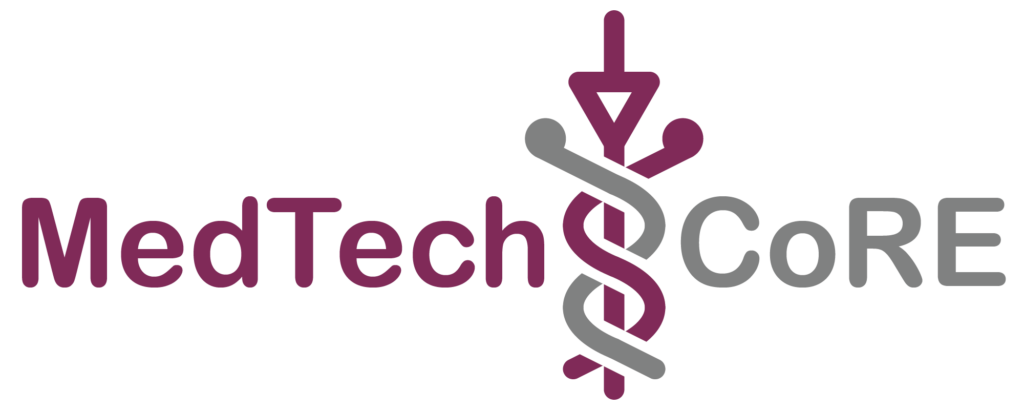

References
- Zhaohan Xiong, Qing Xia, Zhiqiang Hu, Ning Huang, Cheng Bian, Yefeng Zheng, Sulaiman Vesal, Nishant Ravikumar, Andreas Maier, Xin Yang, Pheng-Ann Heng, Dong Ni, Caizi Li, Qianqian Tong, Weixin Si, Elodie Puybareau, Younes Khoudli, Thierry Géraud, Chen Chen, Wenjia Bai, Daniel Rueckert, Lingchao Xu, Xiahai Zhuang, Xinzhe Luo, Shuman Jia, Maxime Sermesant, Yashu Liu, Kuanquan Wang, Davide Borra, Alessandro Masci, Cristiana Corsi, Coen de Vente, Mitko Veta, Rashed Karim, Chandrakanth Jayachandran Preetha, Sandy Engelhardt, Menyun Qiao, Yuanyuan Wang, Qian Tao, Marta Nuñez-Garcia, Oscar Camara, Nicolo Savioli, Pablo Lamata, Jichao Zhao. "A Global Benchmark of Algorithms for Segmenting the Left Atrium from Late Gadolinium-Enhanced Cardiac Magnetic Resonance Imaging." Medical Image Analysis 67 (2020): 101832.
- Christopher McGann, Nazem Akoum, Amit Patel, Eugene Kholmovski, Patricia Revelo, Kavitha Damal, Brent Wilson, Josh Cates, Alexis Harrison, Ravi Ranjan, Nathan S. Burgon, Tom Greene, Dan Kim, Edward V.R. DiBella, Dennis Parker, Rob S. MacLeod, Nassir F. Marrouche. "Atrial Fibrillation Ablation Outcome is Predicted by Left Atrial Remodeling on MRI." Circulation: Arrhythmia and Electrophysiology 7, no. 1 (2014): 23-30.
- Brian J. Hansen, Jichao Zhao, Thomas A. Csepe, Brandon T. Moore, Ning Li, Laura A. Jayne, Anuradha Kalyanasundaram, Praise Lim, Anna Bratasz, Kimerly A. Powell, Orlando P. Simonetti, Robert S.D. Higgins, Ahmet Kilic, Peter J. Mohler, Paul M.L. Janssen, Raul Weiss, John D. Hummel, Vadim V. Fedorov. "Atrial Fibrillation Driven by Micro-Anatomic Intramural Re-Entry Revealed by Simultaneous Sub-Epicardial and Sub-Endocardial Optical Mapping in Explanted Human Hearts." European heart journal 36, no. 35 (2015): 2390-2401.
- Jichao Zhao, Brian J. Hansen, Yufeng Wang, Thomas A. Csepe, Lidiya V. Sul, Alan Tang, Yiming Yuan, Ning Li, Anna Bratasz, Kimerly A. Powell, Ahmet Kilic, Peter J. Mohler, Paul M. L. Janssen, Raul Weiss, Orlando P. Simonetti, John D. Hummel, Vadim V. Fedorov. "Three‐dimensional Integrated Functional, Structural, and Computational Mapping to Define the Structural “Fingerprints” of Heart‐Specific Atrial Fibrillation Drivers in Human Heart Ex Vivo." Journal of the American Heart Association 6, no. 8 (2017): p.e005922.
- Zhaohan Xiong, Vadim V. Fedorov, Xiaohang Fu, Elizabeth Cheng, Rob Macleod and Jichao Zhao, "Fully Automatic Left Atrium Segmentation from Late Gadolinium Enhanced Magnetic Resonance Imaging Using a Dual Fully Convolutional Neural Network." IEEE Transactions on Medical Imaging 38, no. 2 (2018): 515-524.